What marketers need to know now to navigate modern search interfaces.
A lot has been written about how generative AI will forever change the way we search and source information on the web.
Much less has been written about practical actions marketers can take to future-proof their content in the age of large language models (LLMs) and personal AI agents.
But before we get into the details on optimizing content for LLMs, or as some call it, Large Language Model Optimization (LLMO), let’s check in on where we’re currently at with LLMs and AI-powered search engines overall.
Where are we at with LLMs and search engines utilizing this technology?
- OpenAI originally announced SearchGPT in July 2024. The app came out of beta at the end of October and will be rolled out first to premium subscribers and enterprise users, followed by the general public.
- Perplexity is an AI answer engine that launched back in 2022, with a newer enterprise launching in April 2024. It reportedly has over 10 million active monthly users.
- Google AIO (AI overviews, powered by Gemini) is Google’s embedded LLM technology, currently in public beta in most countries around the world.
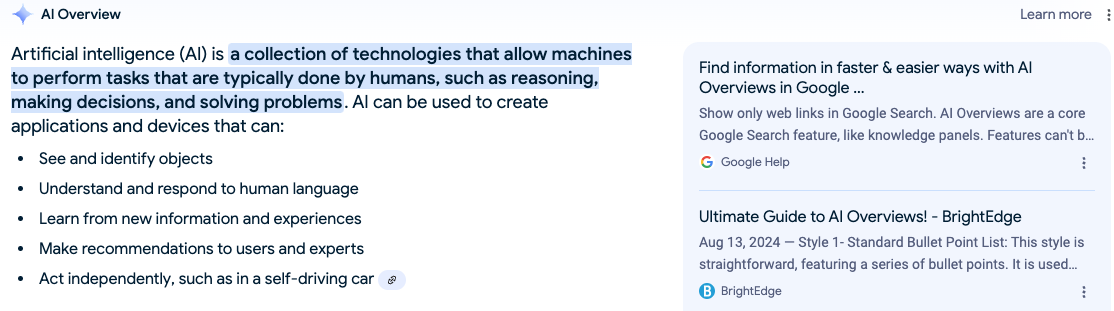
Is AI going to affect my SEO traffic?
This is a complex question with many variables. The short answer is, ‘it probably is’ but is highly dependent on the search query and product you’re selling.
That said, there are many similarities between creating helpful content so it’s ranked highly by traditional search engines, like Google, and also so it can be crawled and found by LLMs.
When discussing organic traffic gains or losses, nuance is important. There is a difference between traditional search results vs. AIOs within a single search interface, such as Google, and Google vs. other generative AI search engines altogether, like Perplexity.
One in-depth study by Rand Fishkin over at SparkToro around the media headlines surrounding Google’s imminent demise actually found that Google is not losing market share and is in fact, stronger than ever.
Within Google search itself, so far, AIOs include citations (links) to sites that also appear in top organic search result placements. So if you can manage to get onto page 1 of the SERP, chances are your brand will also appear in AIOs for similar queries.
The age of generative AI provides an opportunity for brands to focus on the entire customer journey to ensure their content is truly helpful during all stages, especially at point of conversion. Given generative AI’s predominant role as an ‘answer engine,’ customers still require depth of information that AIOs can’t currently solve for. This is especially true for high ticket items like travel.
At Dune7, we’re currently helping clients to decipher search intent and rank higher for commercial terms, versus just informational or navigational ones, the latter of which may come under more pressure from AI generated search results.
Lastly, in the case of Google’s AIOs, this ‘top of page real estate’ is not dissimilar to rich snippets that have been around for years.
Prior to generative AI, if you conducted a search for “how old is Paul McCartney,” Google would give you the answer right in the SERP; no need to click. Again, the intent behind an informational search such as this is that a user requires a quick answer, versus an in depth article.
This is the basic UX of AIOs that are triggered predominantly by “informational” type queries, as opposed to “commercial” ones.
Let’s look at the similarities between large language model optimization (LLMO) and search engine optimization (SEO)
Both LLMO and SEO share foundational principles centered around understanding user intent and creating content that resonates with target audiences. Here are some key similarities:
- User Intent: Just as SEO revolves around deciphering what users are searching for, LLMO focuses on understanding the queries and prompts that users present to language models. Brands must consider how their content aligns with the types of questions consumers are likely to ask.
- Keyword Relevance: To this day, SEO relies on the strategic placement of keywords and phrases into content that potential customers are searching for. Similarly, LLMO involves integrating relevant phrases and concepts that an LLM might recognize and respond to, enhancing the likelihood of your content being highlighted in AI-driven searches.
- Content Quality: High-quality content has always been paramount for SEO success, and this principle applies to LLMO as well. LLMs prioritize coherent, contextually rich, and informative content, meaning brands must prioritize depth and accuracy in their messaging.
Learn more about how Dune7 helps brands create high quality content through our proprietary Customer First Search framework.
- Structured Data: Just as SEO utilizes structured data to help search engines understand content, LLMO benefits from clear entity markup. In fact, given the tendency for AI to “hallucinate” responses, schema markup can help LLMs to understand the content, and context, on your web pages.
- Engagement Metrics: Both SEO and LLMO are influenced by user engagement. In SEO, metrics like click-through rates and dwell time matter; in LLMO, user feedback and interactions can influence how content is perceived and ranked by AI platforms.
That said, there are a few key differences between SEO and LLMO
Before we get into the details on how SEO and LLMO differ, it’s worth it to have a surface-level understanding of how search engines, like Google, and LLMs, like ChatGPT, work to return answers to user queries.
Google’s algorithm has been developed over decades, and is still constantly evolving. But, at its core, Google search contains three major elements:
- Crawling: the process by which a search engine, in Google’s case, googlebot, visits and downloads content from URLs.
- Indexation: the search engine analyzes the text, images, and video files on the page, and stores the information in the index, which is a large database.
- Serving search results: this is the part that users see, the actual search results themselves. Google’s algorithm contains 200+ ranking factors from backlinks to perceived content relevance.
Large language models, on the other hand, operate somewhat differently. Here’s how LLMs work, at a basic level:
- Training: LLMs are trained on huge amounts of data, oftentimes, via crawling web pages in a similar fashion as traditional search engines.
- Deep learning: a type of advanced machine learning, deep learning allows LLMs to understand how words and sentences fit together. This involves probabilistic analysis of unstructured data which then helps the model to recognize differences and similarities between pieces of content without human intervention.
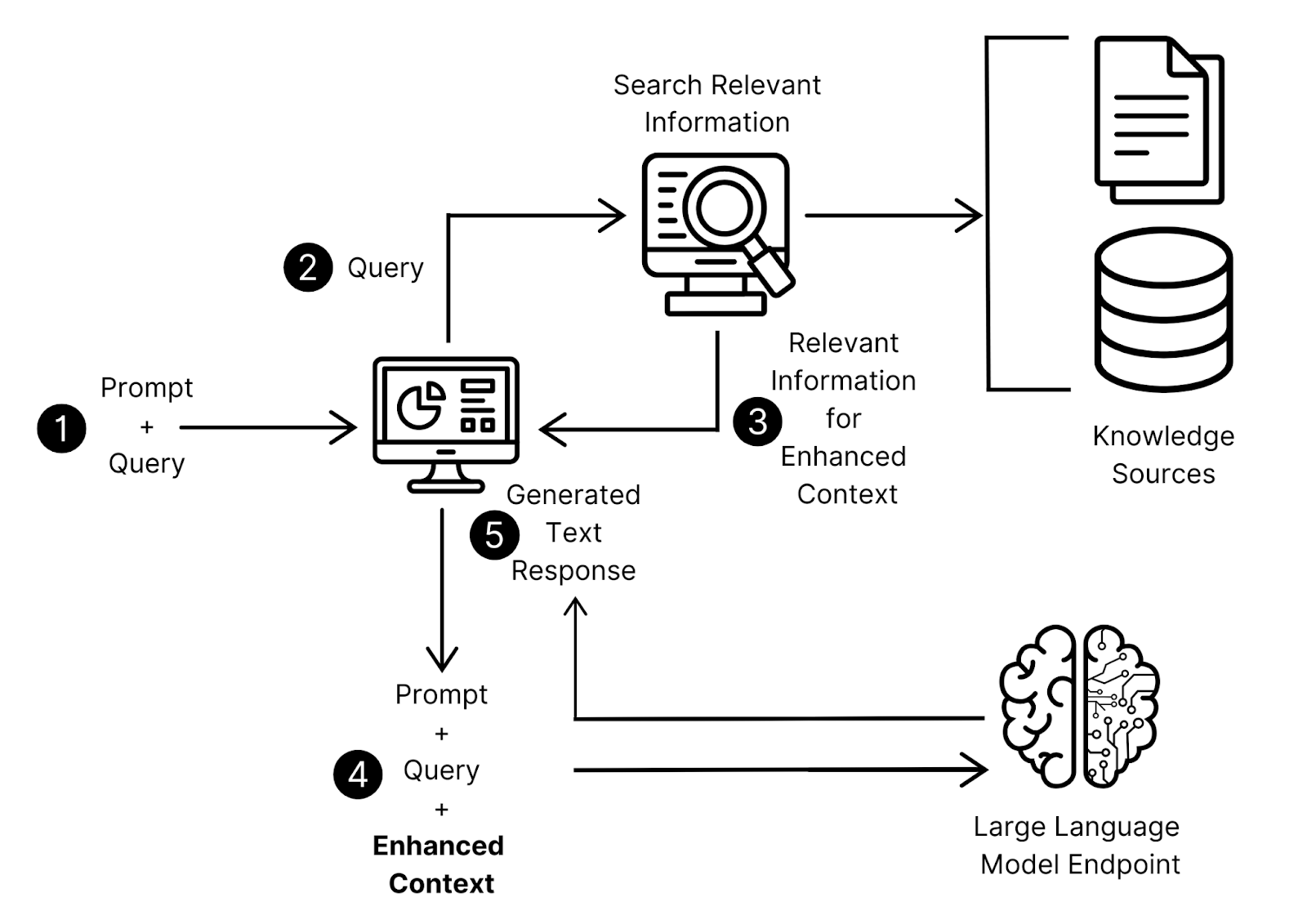
- Prompt-tuning: once the LLM has ingested data, it needs to be fine-tuned to the specific task for which it will be focused on. For example, in the case of a generative AI model, interpreting questions and generating responses.
- Answer generation: similar to traditional search engines, the last step is the piece users see, which is the output or response to the prompt.
What are the differences between SEO and LLMO?
1. Search Intent vs. Conversational Context
- SEO: Focuses on optimizing content for specific keywords or search queries that users input into search engines like Google. It’s all about ranking for targeted keywords, phrases, and simple questions.
- LLMO: Optimizes content for natural language, conversation-like responses, and deeper context. LLMs aim to understand nuanced queries, offering answers that may span several topics or multi-part questions.
What to do differently:
- Brands need to structure content in a way that can be easily broken down and recombined into responses to complex or multi-layered questions.
- Prioritize semantic depth over keyword density. This means focusing on the meaning and substance of a specific topic rather than simply targeting specific words.
- Content and URL mapping becomes even more important; make sure you have pages mapped to all points in your user journey and also be mindful of pages that might cannibalize, or compete with each other, on the same topic.
2. Content Structuring
- SEO: Focuses on optimizing for clear headings, proper use of metadata, keyword density, and technical aspects like site speed or mobile-friendliness.
- LLMO: Large language models extract information without needing structured markup, although as discussed above, schema markup could help. Clear, logically organized content still helps models understand and present content accurately.
What to do differently:
- Write more concise, informative answers that directly respond to potential user inquiries. Incorporate FAQs, summaries, and structured snippets that an LLM can easily parse and extract for use in responses.
- Use tools like Google’s ‘People Also Ask’ section to find out the actual questions your audience is asking on a given topic.
- Use schema markup to aid search engines and models alike, but realize LLMs focus less on technical SEO elements and more on the quality and utility of the content itself.
3. Backlinks vs. Contextual Authority
- SEO: Backlinks and domain authority still play a key role in ranking pages on Google. Earning high-quality backlinks from authoritative sites is critical for improving visibility.
- LLMO: Backlinks are less relevant for LLMs. Instead, these models prioritize the relevance and depth of information. The focus is on providing the most contextually appropriate and informative answers based on the model’s training data.
What to do differently:
- Brands need to shift toward building contextual authority by creating in-depth content that answers questions comprehensively.
- Prioritize on-page SEO and topic clustering/content hubs using the E-E-A-T framework.
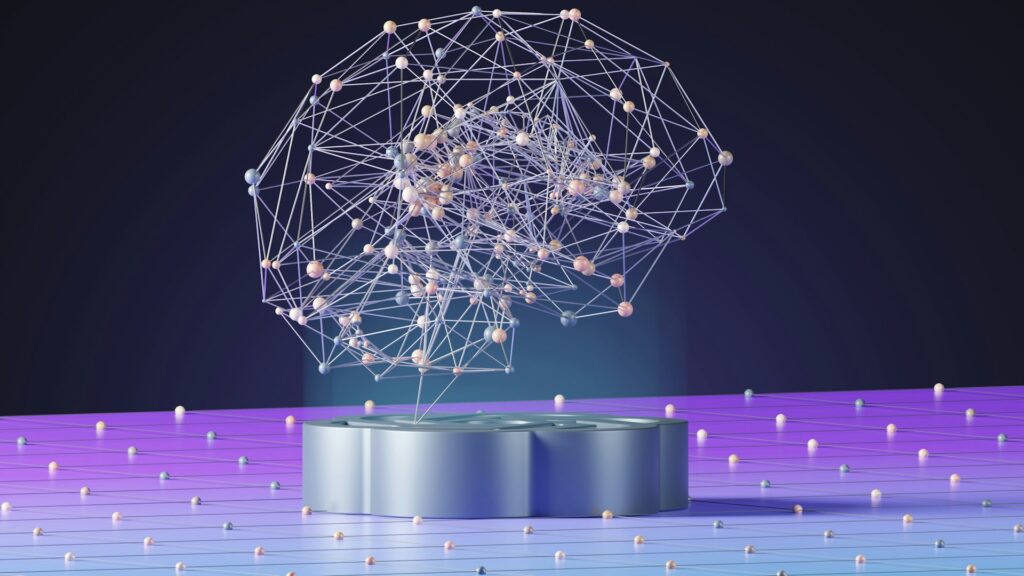
4. User Experience (UX)
- SEO: Google and other search engines weigh UX elements, such as page load speed, mobile friendliness, and ease of navigation, to rank pages.
- LLMO: While these models don’t "see" a website like a traditional user or search engine crawler, the readability and clarity of the content matter because the LLM will extract and summarize information for users.
What to do differently:
- Ensure content is easy to consume—breaking down large blocks of text, simplifying language where appropriate, and focusing on delivering information in manageable, digestible blocks.
5. Content Length
- SEO: Long-form content often ranks well because it can cover a topic thoroughly and provides ‘information gain,’ offering users new and fresh perspectives on a topic.
- LLMO: Length is less critical compared to conciseness and relevance. LLMs prioritize content that efficiently provides clear and accurate answers.
What to do differently:
- Aim for a balance between depth and brevity. While comprehensive content is necessary, focus on clear, direct answers that AI models can summarize easily.
Bringing it all together
As Large Language Models continue to reshape the digital landscape, brands must adapt, just as they did at the dawn of the mobile era. By drawing parallels with well-established SEO practices and implementing targeted LLMO strategies, marketers can enhance their visibility and maintain ROI for channels such as search.
While Google Search Console unfortunately doesn’t break out keyword and click data by AIO vs. traditional search results, platforms such as Ahrefs are already adapting to show marketers keywords that trigger AIOs.
At the end of the day, SEO and LLMO are quite similar in that both require the ongoing optimization of content so it can be found by customers.
No comments.